Graph processing with Pregel
What is Pregel ?
Pregel is a distributed graph processing system developed by Google that follows a vertex-centric approach for large-scale graph computations.
How it works ?
It works through iterative supersteps where each vertex:
- Receives messages from the previous superstep
- Performs computations based on received messages
- Sends messages to neighboring vertices for the next superstep
Here's a simple example of how Pregel would compute the shortest path from a source vertex:
# Vertex Program pseudocode
def compute(vertex, messages):
if superstep == 0:
if vertex.id == source_vertex:
vertex.value = 0
send_to_neighbors(vertex.value + 1)
else:
vertex.value = INFINITY
else:
min_distance = min(messages)
if min_distance < vertex.value:
vertex.value = min_distance
send_to_neighbors(vertex.value + 1)
vote_to_halt()
The process continues until no more messages are being sent (convergence).
Key Features
Key features of Pregel include:
- Fault tolerance through checkpointing
- Synchronous superstep execution
- Message passing between vertices
- Ability to handle very large graphs through distribution
This model has inspired many modern graph processing systems like Apache Giraph and GraphX.
Lambda Architecture
Storm Architecture
Apache Storm is a distributed real-time stream processing system designed for big data. Here's a simple way to understand and remember its architecture:
Core Components
- Spouts: Think of these as "water sources"
- Entry points that emit data streams
- Like a tap that continuously releases water
- Bolts: Think of these as "processors"
- Transform, filter, or aggregate data
- Like filters or treatment stations in a water system
Topology
Remember it as a "flowing river system": Data flows from Spouts through various Bolts, creating a directed graph (topology) – just like how rivers flow through different processing points.
graph LR
A["Spout"] --> B["Bolt 1"]
B --> C["Bolt 2"]
B --> D["Bolt 3"]
C --> E["Final Bolt"]
D --> E
Memorization Tip: Think of it as a water treatment system: Source (Spout) → Processing Plants (Bolts) → Clean Water (Results)
YARN
MapReduce 1.0 Architecture
In MapReduce 1.0, JobTracker was the central component responsible for both resource management and job scheduling/monitoring. This created a bottleneck as it had to handle all responsibilities:
- Resource Management: Tracking resource availability across nodes
- Job Scheduling: Assigning tasks to nodes
- Job Monitoring: Tracking job progress and handling failures
YARN (Yet Another Resource Negotiator)
YARN decouples the resource management and job scheduling functions, creating a more scalable system:
- Resource Manager (RM): Handles cluster-wide resource allocation
- Node Manager (NM): Manages resources on individual nodes
- Application Master (AM): Manages specific application lifecycle
YARD decoupled Resource Management from MR1 :
YARN uses an API that allows different Big Data frameworks like MapReduce 2 and Spark to request resources from the central YARN resource manager
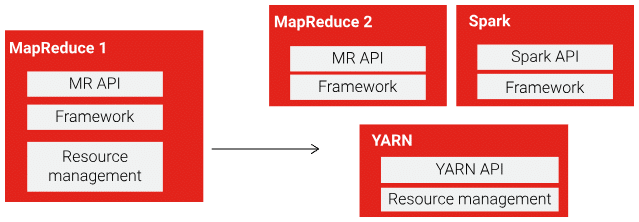
MapReduce 2.0 with YARN
MapReduce 2.0 runs as a YARN application, with better scalability and resource utilization:
- Each MapReduce job gets its own Application Master
- Resource Manager handles only resource allocation
- Supports multiple applications beyond just MapReduce
graph TD
subgraph "MapReduce 1.0"
JT[JobTracker] --> TT1[TaskTracker 1]
JT --> TT2[TaskTracker 2]
end
subgraph "YARN (MapReduce 2.0)"
RM[Resource Manager] --> NM1[Node Manager 1]
RM --> NM2[Node Manager 2]
RM --> AM[Application Master]
AM --> NM1
AM --> NM2
end
%%Diagram shows the architectural difference between MR1 and YARN
The key advantage of YARN is its ability to support multiple distributed computing paradigms beyond MapReduce, making it a more versatile resource management platform for modern big data applications.
MapReduce VS YARN
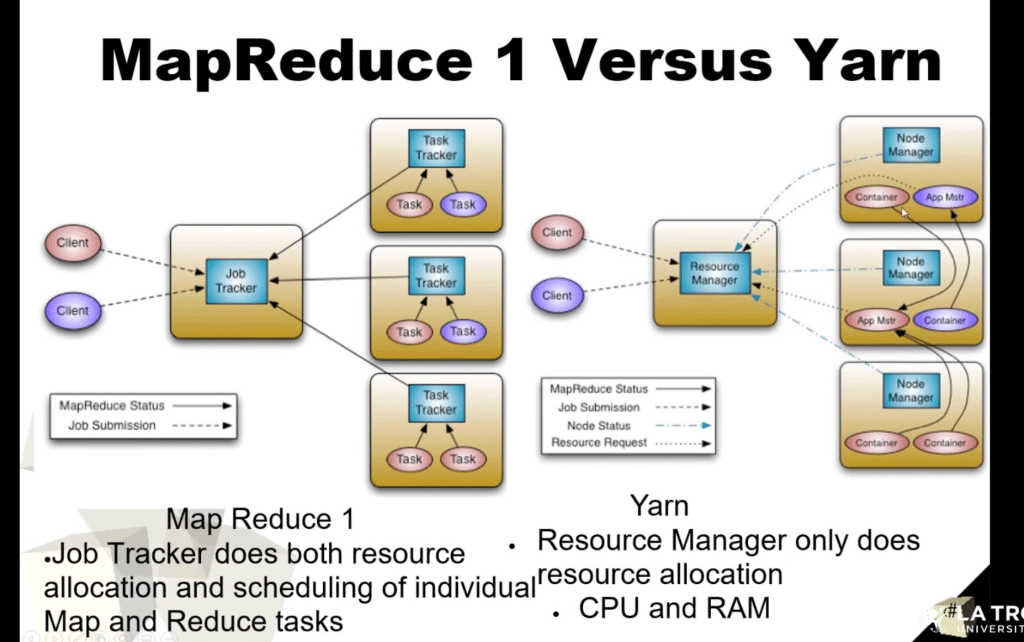
Let me explain the key differences between MapReduce 1.0 and YARN architectures shown in the image and page:
MapReduce 1.0:
- Uses a centralized JobTracker that handles both resource management and job scheduling
- TaskTrackers on each node execute the assigned tasks
- This creates a bottleneck since JobTracker has to manage all responsibilities
YARN (MapReduce 2.0):
- Decouples resource management from job scheduling for better scalability
- Resource Manager (RM) only handles cluster-wide resource allocation
- Node Managers handle resources on individual nodes
- Each application gets its own Application Master to manage its lifecycle
The key advantage is that YARN can support multiple distributed computing frameworks beyond just MapReduce, making it more versatile for modern big data applications.
Node Manager vs Application Master
Node Manager:
- Runs on each node in the cluster
- Manages and monitors resources (CPU, memory, disk, network) on its node
- Reports resource usage to Resource Manager
- Manages containers running on its node
- Handles start/stop/cleanup of containers
Application Master:
- One per application (job-specific)
- Negotiates resources with Resource Manager
- Coordinates application execution flow
- Monitors task progress and handles failures
- Works with Node Managers to execute tasks in containers
In simple terms, Node Manager is like a local supervisor managing resources on one machine, while Application Master is like a project manager coordinating the entire application across multiple nodes.
Examples of Application Master Jobs
1. MapReduce Jobs:
- Manages word count applications across multiple nodes
- Coordinates log analysis tasks by distributing log chunks to different nodes
- Handles large-scale data sorting operations
2. Spark Applications:
- Manages machine learning model training across the cluster
- Coordinates real-time streaming data processing
- Handles interactive data analytics queries
3. Resource Management Tasks:
- Monitors memory usage of individual containers
- Handles task failures by requesting new containers from Resource Manager
- Optimizes data locality by requesting specific nodes for tasks
For example, in a word count MapReduce job, the Application Master would:
- Request containers from Resource Manager based on input data size
- Coordinate with Node Managers to launch map tasks on nodes with data
- Monitor progress of map tasks processing text chunks
- Coordinate the shuffle phase to move data between nodes
- Launch reduce tasks to aggregate final word counts
- Handle any failed tasks by requesting new containers
graph TD
Client["Client (Word Count Job)"] --> AM["Application Master"]
AM --> RM["Resource Manager"]
RM --> NM1["Node Manager 1"]
RM --> NM2["Node Manager 2"]
subgraph "Map Phase"
NM1 --> M1["Map Task 1<br/>Count words in chunk 1"]
NM1 --> M2["Map Task 2<br/>Count words in chunk 2"]
NM2 --> M3["Map Task 3<br/>Count words in chunk 3"]
end
subgraph "Reduce Phase"
M1 --> R1["Reduce Task 1<br/>Aggregate counts A-M"]
M2 --> R1
M3 --> R1
M1 --> R2["Reduce Task 2<br/>Aggregate counts N-Z"]
M2 --> R2
M3 --> R2
end
AM --> |"Monitor Progress"| M1
AM --> |"Monitor Progress"| M2
AM --> |"Monitor Progress"| M3
AM --> |"Monitor Progress"| R1
AM --> |"Monitor Progress"| R2
%%Diagram shows how a MapReduce word count job interacts with YARN components
This diagram illustrates how a word count MapReduce job flows through the YARN architecture:
- Client submits the word count job to Application Master
- Application Master requests resources from Resource Manager
- Resource Manager allocates containers on Node Managers
- Map tasks process individual chunks of text data
- Reduce tasks aggregate the word counts by ranges
- Application Master continuously monitors all tasks and handles failures